In the 1980s, organizations began using multiple databases, online transaction processing databases (OLTP). Data sources scattered across systems in order to store business information. During this time, IBM researchers Barry Devlin and Paul Murphy coined the “business data warehouse.” It came into the picture as the need for data integration intensified. So, It became the standard process of handling data—typically structured—with the arrival of data warehouses. It is done to “extract” data from multiple independent sources, “transform” it into a standard warehouse format, and “load” it in an on-premise warehouse. This single source of truth data is then used for analysis and business decisions. In this blog, we will understand exactly WHAT IS ETL?
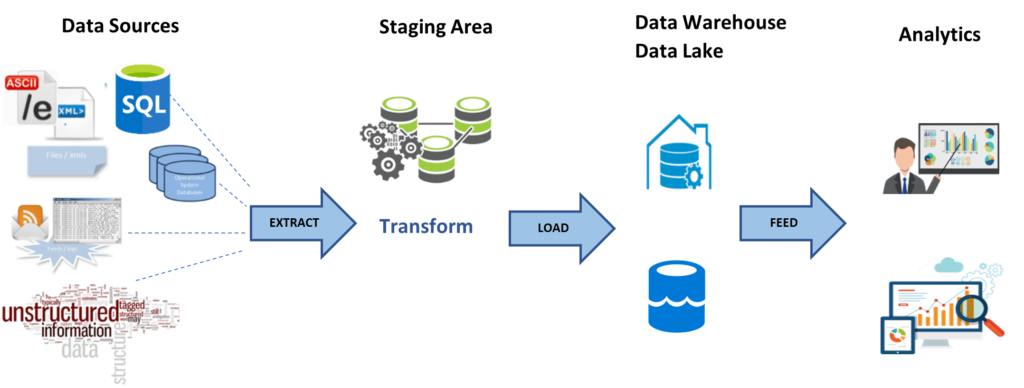
Extract
It is responsible for extracting data from multiple data sources. It held in temporary storage—staging area. Data not conforming to the data warehouse validation rules is not loaded during data extraction and is further investigated to discover the problem.
Transform
It is responsible for making the data values and structures consistent. Hence, The data is transformed to make it suitable for the intended analytical use. Data from multiple source systems are converted in order to a single system format. It may also include data validation, data accuracy, conversion, profiling, and transformation.
Load
It is responsible for moving the transformed data into the target database. It is performed periodically in order to keep the data warehouse updated.
Traditional ETL Falls Short
- Over time, the generated data increased to enormous volumes. so, IDC states that 1.2 zettabytes of new data were created in 2010, which increased to 64.2 zettabytes in 2020. The rapid data growth posed challenges in scaling on-premise data warehouses.
- Typical this process has scheduled batches. hence real-time data processing is not possible.
- We have an upsurge in the generation of semi-structured and unstructured data. Considering since the traditional ETL process could not handle.
- Any changes in the ETL plan caused changes in the data mapping for transformation and reload all the data.
The traditional ETL process changed to handle these issues. Here we welcome modern ETL!
- Modern ETL tools are flexible and can work with structured and unstructured data.
- Modern ETL tools integrate with on-premise environments and cloud data warehouses like Google cloud, Amazon RedShift, snowflake, Microsoft Azure, etc., which support parallel processing and can be easily scaled.
- They are designed in order to move data in real-time and make cutting-edge analytics possible.
- Data lakes and cloud adoption led to flexibility in data transformation—ELT. We will cover this in detail.
Useful Links – CBDA certification training | Data visualization course with Tableau | Power BI Certification Course
Shifting the “T” in ETL
ELT is an alternative to ETL, where the data transformation is pushed down in order to the target database. So, when the data is needed, the transformation process is defined based on the end goal.
Let us explore the differences:
Traditional ETL | Modern ELT |
---|---|
Only relevant and required data fields from multiple sources, usually structured datasets, are loaded into the warehouse. | For instance, Almost all data from the source systems, particularly high-volume, structured and unstructured datasets, are continuously pushed to the data lake or cloud data warehouse. |
Transformations are completed before loading into the data warehouse. | The loaded data is transformed as and when needed for the analysis. |
The transformation process can take a long time, but analytics is fast once the data is transformed. | The transformation process is done only on the required subset of the data with separate resources. Hence, transformation is fast, but analytics can take time if there is not enough processing power. |
This process typically takes place during off-traffic hours in batches and is not flexible. Hence, real-time processing is not possible. | A flexible and real-time analysis is possible as data from the source systems are pushed in real-time. |
If the transformed data does not work for the business use case, or if more data are required, re-work from the first step is needed. | ELT gives organizations the ability in order to transform data on the fly and accommodate changes. |
Information can be lost when raw data is transformed for a specific use case. | Untransformed data can be reused for different goals. |
Current Uses of ETL
ETL is commonly used in different ways like:
- Traditional ETL: A data warehouse stores data from multiple sources and is analyzed for business purposes. This enables executives, managers, and stakeholders in order to make data-driven decisions.
- ETL for Data Quality: Good data quality is imperative for good insights. Tools are used for data cleansing, profiling, and auditing. They can also be integrated into other data cleaning tools to ensure that the best data quality is available for decision-making.
- ETL and Big Data: Data generated today is a mix of structured, unstructured, and semi-structured, and the massive volume of data generated. hence, This cannot be handled in the traditional warehouse. so, Hadoop, an open-source, scalable platform, and many others allow the processing of large datasets, and is used in order to load and convert data into Hadoop. Since, Good quality, real-time data can be made available through ETL for data scientists who build machine learning and Artificial Intelligence models.
- ETL for IoT: For instance, IoT is a collection of devices that can collect and transmit data through the sensors embedded in equipment hardware like smartphones, wearable devices, etc. It helps move data from multiple IoT sources to a single place for analysis.
- ETL for Database Replication: ETL copies data from source databases—like Oracle, Microsoft SQL Server, PostgreSQL, MongoDB, or others—into the cloud data warehouse, Once copied, it is updated for changes.
- ETL for Cloud Migration: Many organizations are moving to the cloud for scalability, higher security, and cost-effectiveness. These tools facilitate this migration from on-premise to cloud.
ETL Use Cases
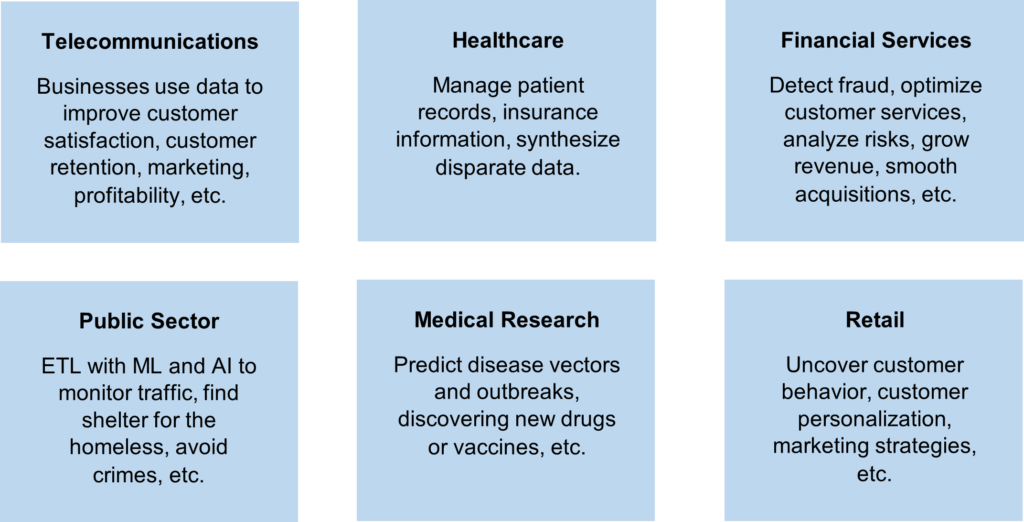
ETL Tools
They are used to save time and money and eliminate the need for writing code when a new data warehouse is developed. As a result, Tools allow automation and ease of use with visual drag-drop, complex data management, and security. for instance, The variety of tools includes commercial, open-sourced, cloud-based, and/or on-premise tools. As a result, The decision of which tool is suitable depends on the data and business requirements.
Commercial Tools
- Informatica PowerCenter: End-to-end data management platform that connects, manages and unifies data across any multi-cloud using AI.
- Oracle – Data Integrator: Data integration platform that all data integration requirements, including big data support and added parallelism.
- Microsoft – SQL Server Integrated Services (SSIS): It is a platform for building enterprise-level data integration and transformation solutions.
- AWS Glue: For instance, It is a server less data integration service that makes it easy to discover, prepare, and combine data for analytics, machine learning, and application development.
- Xplenty: It is a cloud-based ETL & ELT tool for Big data analysis with low coding requirements, data security, and customization.
Many other tools are available like SAS – Data Integration Studio, SAP Data Integrator, Ab Initio, IBM Infosphere Information Server, Alooma, and many more.
Open-Source Tools
- Pentaho: It is an ETL tool run by Kettle runtime, where the procedures are saved in XML and interpreted in Java files during data transformation.
- Talend – Talend Open Studio for Data Integration: This tool provides data integration with strong connectivity, easy adaptability, and good extraction and transformation process flow.
- CloverETL: It is based on the Java platform. You can manage the entire lifecycle of a data pipeline from design, deployment to evolution and testing.
- Stitch: Along with ETL, this cloud-based platform gives the power in order to secure, analyze, and govern data by centralizing it into the infrastructure.
You can also choose to write Extract, Transform, Load scripts on your own using SQL or python using available open-source frameworks, libraries, and tools.
Useful Links – CBDA certification training | Data visualization course with Tableau | Power BI Certification Course
ETL Benefits
- It makes possible historical analysis of data for businesses using legacy and new data.
- Extract, Transform, Load helps with data collection, cleansing, synchronization, profiling, and auditing. This ensures data is trustworthy to uncover meaningful insights for business decisions.
- It acts as a single source of truth for good quality data available for end-users.
- Supports data governance that includes data reusability, security, and integrity.
- Automating the process using tools saves coding effort, time, and cost.
ETL Challenges
- Extracting data from multiple systems and identifying the desired subset of data from various sources may be tedious. Scaling up as business data grows is challenging.
- High-volume data movement through Extract, Transform, Load requires a lot of planning and effort to maintain consistency. Validation of the incoming data may be tedious and time-consuming.
- Defining correct transformation rules in order to ensure that accurate source data is correctly loaded in the target tables.
- For incremental loads, comparing new data with the existing data poses many challenges.
- Not suitable for real-time decision-making.
Building an ETL Strategy
Today, businesses face fierce competition, and they have to carefully plan how they can use Extract, Transform, Load most effectively.
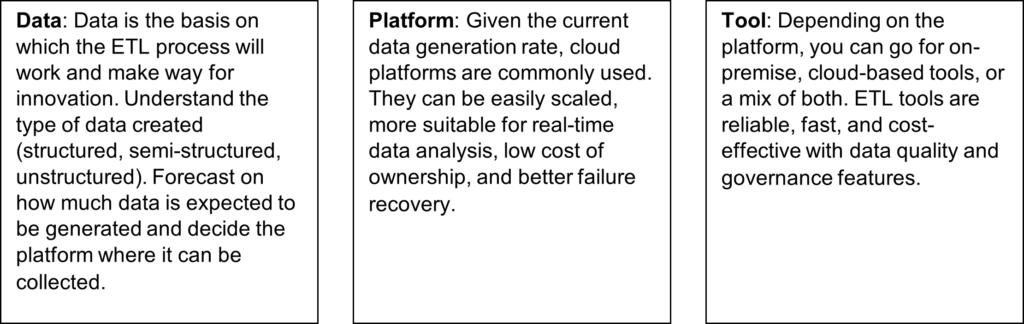
Future of ETL
As data continues in order to grow, the future of Extract, Transform, Load will work around handling vast amounts of data of different types.
- Develop advanced cloud Extract, Transform, Load tools in order to handle more variety of data faster and in real-time.
- Prepare data for Machine Learning and Artificial Intelligence as they continue to advance.
- Making data readily available to all users irrespective of their technical background. So, Encouraging everyone to use data for analysis to drive data culture in organizations.
References
https://www.matillion.com/what-is-etl-the-ultimate-guide/#future
https://www.alooma.com/blog/category/etl
https://www.sas.com/en_us/insights/data-management/what-is-etl.html
http://www.databaseetl.com/etl-tools-top-10-etl-tools-reviews/
https://www.qlik.com/us/etl/etl-vs-elt
About Techcanvass
Techcanvass is an IT training and consulting organization. We are an IIBA Canada Endorsed education provider (EEP) and offer business analysis certification courses for professionals.
We offer CBDA certification training to help you gain expertise in Business Analytics and work as a Business Analyst in Data Science projects.
You can also learn Data visualization skills by joining our Data visualization course with Tableau and Power BI Certification Course.